spot price的問題,我們搜遍了碩博士論文和台灣出版的書籍,推薦Esposito, Dino寫的 Programming ML.Net 和Miller, Judith的 Miller’’s Collectibles Handbook & Price Guide 2021-2022都 可以從中找到所需的評價。
這兩本書分別來自 和所出版 。
國立高雄大學 金融管理學系碩士班 王文楷所指導 林冠穎的 依投資組合價值調整之簡單Delta避險策略 : 以台指選擇權為例 (2021),提出 spot price關鍵因素是什麼,來自於選擇權避險。
而第二篇論文國立臺灣科技大學 企業管理系 呂志豪、鄭仁偉所指導 許子敬的 以機器學習模型結合市場資訊之價格預測系統 - 以半導體市場為例 (2021),提出因為有 機器學習、深度學習、多層感知機、記憶體、價格預測的重點而找出了 spot price的解答。
Programming ML.Net
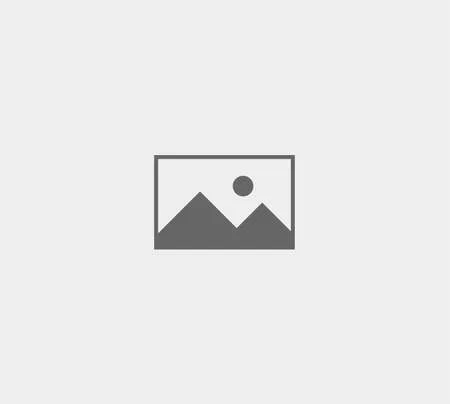
為了解決 spot price 的問題,作者Esposito, Dino 這樣論述:
The expert guide to creating production machine learning solutions with ML.NET! ML.NET brings the power of machine learning to all .NET developers-- and Programming ML.NET helps you apply it in real production solutions. Modeled on Dino Esposito’s best-selling Programming ASP.NET, this book take
s the same scenario-based approach Microsoft’s team used to build ML.NET itself. After a foundational overview of ML.NET’s libraries, the authors illuminate mini-frameworks ("ML Tasks") for regression, classification, ranking, anomaly detection, and more. For each ML Task, they offer insights for ov
ercoming common real-world challenges. Finally, going far beyond shallow learning, the authors thoroughly introduce ML.NET neural networking. They present a complete example application demonstrating advanced Microsoft Azure cognitive services and a handmade custom Keras network-- showing how to lev
erage popular Python tools within .NET. 14-time Microsoft MVP Dino Esposito and son Francesco Esposito show how to: Build smarter machine learning solutions that are closer to your user’s needsSee how ML.NET instantiates the classic ML pipeline, and simplifies common scenarios such as sentiment anal
ysis, fraud detection, and price predictionImplement data processing and training, and "productionize" machine learning-based software solutionsMove from basic prediction to more complex tasks, including categorization, anomaly detection, recommendations, and image classificationPerform both binary
and multiclass classificationUse clustering and unsupervised learning to organize data into homogeneous groupsSpot outliers to detect suspicious behavior, fraud, failing equipment, or other issuesMake the most of ML.NET’s powerful, flexible forecasting capabilitiesImplement the related functions of
ranking, recommendation, and collaborative filteringQuickly build image classification solutions with ML.NET transfer learningMove to deep learning when standard algorithms and shallow learning aren’t enough"Buy" neural networking via the Azure Cognitive Services API, or explore building your own wi
th Keras and TensorFlow
spot price進入發燒排行的影片
สกินแคร์ราคานักเรียนนักศึกษามาแล้วววว ทุกตัวในนี้ใช้ดีจริง คัดมาให้แต่ตัวปังๆ เศรษฐกิจไม่ดีแต่ผิวเราต้องดี! หวังว่าจะช่วยเซฟเงินทุกคนได้น๊า
(รายละเอียดโปรดักส์กดดูข้างใต้เลยจ้า ??)
.
.
.
OTHER FAVORITE PRODUCTS VIDEOS
FAVORITE SKINCARE 2020
https://youtu.be/OLTp2PjoEwM
FAVORITE MAKEUP 2020
https://youtu.be/dh1D8SraAa0
MIDYEAR FAVORITES 2019
https://youtu.be/8bpMuLy7DIk
BEST SKINCARE 2019
https://youtu.be/emcHb-oKx94
BEST MAKEUP 2019 & TRY-ON
https://youtu.be/wk8h3dwCiJ8
FAVORITE SKINCARE MIDYEAR 2020
https://youtu.be/jaKElNxXPWM
FAVORITE HAIR CARE PRODUCTS
https://youtu.be/tReLo9ptczU
.
.
.
DISCLAIMER: *some are given product but this video is NOT SPONSORED* ทุกตัวมาจากการใช้จริง ผลลัพธ์อาจแตกต่างไปในสภาพผิวของแต่ละคน
Price updated on 1 May 2021
ราคาอัปเดตวันที่ 1 พ.ค. 2564
(อาจมีการคลาดเคลื่อนในอนาคต)
++ CLEANSER
Cetaphil
Gentle Skin Cleanser
125 ml 240 baht ซื้อได้ที่ https://bit.ly/3xEsUef
250 ml 425 baht ซื้อได้ที่ https://bit.ly/3357f0t
Protex
Life CICA Acne Clear
109 baht ซื้อได้ที่ https://bit.ly/335VJlH
++ TONER
SKIN1004
Madagascar Centella Toning Toner
586 baht
ซื้อได้ที่ https://bit.ly/3ePf8gi
Thayers
Rose Petal Witch Hazel Toner
355 ml 599 baht ซื้อได้ที่ https://bit.ly/3e6CLS5
89 ml 290 baht ซื้อได้ที่ https://bit.ly/2SbVpiV
++ ESSENCE
Cosrx
Advanced Snail 96 Mucin Power Essence
570 baht ซื้อได้ที่ https://bit.ly/3nNYGkx
Za
True White Ex Essence Lotion
320 baht ซื้อได้ที่ https://bit.ly/3eHVGBN
Pond’s
White Beauty Super Essence
110 ml 269 baht ซื้อได้ที่ https://bit.ly/3t1PXMx
12 ml 39 baht ซื้อได้ที่ 7-11
++ SERUM
SKIN1004
Madagascar Centella Ampoule
586 baht
ซื้อได้ที่ https://bit.ly/3eLcIPG
Garnier
Light Complete Booster Serum
319 baht ซื้อได้ที่ https://bit.ly/3vxNTgG
Garnier
Sakura White Hyaluron Booster Serum
319 baht ซื้อได้ที่ https://bit.ly/3vyQkj9
Cute Press
Booster Serum
299 baht
สูตรสีเขียว ซื้อได้ที่ https://bit.ly/3vw011N
สูตรสีฟ้า ซื้อได้ที่ https://bit.ly/3e4oYvi
สูตรสีเหลือง ซื้อได้ที่ https://bit.ly/3nzjU5r
++ LOTION
CeraVe
Moisturizing Lotion
88 ml 221 baht ซื้อได้ที่ https://bit.ly/3vxj0sV
473 ml 552 baht ซื้อได้ที่ https://bit.ly/3eNU2il
++ MASK
Mizumi
Sulfur Clay Mask
4g 49 baht ซื้อได้ที่ 7-11
แบบกล่อง 6 ซอง 294 baht ซื้อได้ที่ https://bit.ly/3t0YaAw
True Trust Clinic
Natural Clay Mask
499 baht
fb & ig: @truetrustclinic @aesthlabofficial
Cute Press
Sleeping Mask
279 baht
สูตรสีเขียว ซื้อได้ที่ https://bit.ly/3t8BCO6
สูตรสีฟ้า ซื้อได้ที่ https://bit.ly/3vry0by
สูตรสีเหลือง ซื้อได้ที่ https://bit.ly/3nzcpvn
Faith in Face
Mask Sheet
39 baht
ซื้อได้ที่ https://bit.ly/3nEySHf
++ ACNE
sasi
ACNE Sol 2-in-1 Spot Treatment Gel
49 baht ซื้อได้ที่ https://bit.ly/3xxmddX
MizuMi
Peptide Acne Gel
4g 49 baht ซื้อได้ที่ 7-11
แบบกล่อง 6 ซอง 294 baht ซื้อได้ที่ https://bit.ly/3nz8GxT
Mizumi
B3 Acne Concentrate Serum
6g 49 baht ซื้อได้ที่ 7-11
แบบกล่อง 6 ซอง 294 baht ซื้อได้ที่ https://bit.ly/3xvZizD
++ SUNSCREEN
KAUV Whitening Soft Cream SPF 50+ PA++++
30g 189 baht ซื้อได้ที่ https://bit.ly/330uwAT
แบบกล่อง 6 ซอง 220 baht ซื้อได้ที่ https://bit.ly/3ta2H3w
Cute Press
UV Expert Protection All Day Bright Tone Up Sunscreen SPF 50+ PA++
246 baht ซื้อได้ที่ https://bit.ly/3vvpqZn
L’Oreal
UV Perfect Matte & Fresh Long UVA SPF 50+ PA+++
239 baht ซื้อได้ที่ https://bit.ly/3gS39ks
++ MAKEUP REMOVER
JUV
Micellar Water Anti-Acne Cleanser
299 baht
ซื้อได้ที่ https://bit.ly/3gPWuaq
Maybelline
Eye + Lip Makeup Remover
40ml 99 baht https://bit.ly/3t6A2Of
70ml 159 baht https://bit.ly/2NLumsH
150ml 299 baht (x2 449 baht ซื้อได้ที่ https://bit.ly/3e6aCuv)
SoftNe’
Makeup Remover Micellar Water
293 baht ซื้อได้ที่ https://bit.ly/3e89sOY
SoftNe’
Makeup Remover Wipes
สูตร Acne Care
ห่อ 12 แผ่น 60 baht ซื้อได้ที่ https://bit.ly/3e2xyL7
ห่อ 20 แผ่น 129 baht ซื้อได้ที่ https://bit.ly/3xFkAuv
สูตร Grape Seed Oil
ห่อ 12 แผ่น 60 baht ซื้อได้ที่ https://bit.ly/3aSOhyC
ห่อ 20 แผ่น 129 baht ซื้อได้ที่ https://bit.ly/3aS2z2r
.
.
.
CONTACT LENS: Idollens Roze Beige Brown
SHIRT: Zara
VEST: CHUU Korea
.
.
.
CAMERA:
Canon RP Lens RF 24-105mm
Editing Software: Final Cut Pro
Music:
BGM President - Brunch II
Daystar - Cute
Lukrembo - Teapot
INSTAGRAM: https://www.instagram.com/babyjingko
FACEBOOK: https://facebook.com/babyjingko
TWITTER: https://twitter.com/babyjingko
__________________________________________
依投資組合價值調整之簡單Delta避險策略 : 以台指選擇權為例
為了解決 spot price 的問題,作者林冠穎 這樣論述:
本文旨在探討選擇權之delta避險策略,主要討論之避險策略為依投資組合價值調整之避險策略(value-based),其調整方式是依照投資組合價值變動比率進行調整。首先利用蒙地卡羅法模擬標的價格,並對提出依投資組合價值調整之避險策略和依時間調整之避險策略(time-based)進行測試,接著再以台灣加權股價指數選擇(TXO)進行實證測試,而本文對避險績效的評估指標是避險誤差以及避險誤差之標準差,另外對交易成本的評估指標為現貨部位之調整價值與調整次數。本研究之結果,在蒙地卡羅法的避險測試之結果顯示,本文策略在適當的調整比率下,避險績效可以達到與依時間調整之避險策略相同水準,而本文策略又有更低的交
易成本,因此可以說明本文策略優於依時間調整之避險策略;在實證結果中顯示,在一些調整比率下的本文策略能夠在避險績效與交易成本的指標上都勝過依時間調整之避險策略。綜觀以上研究結果,期望本篇研究對需要利用選擇權避險的投資人能有所幫助,並對delta避險策略之發展有所貢獻。
Miller’’s Collectibles Handbook & Price Guide 2021-2022

為了解決 spot price 的問題,作者Miller, Judith 這樣論述:
The brand new, up-to-date guide to the collectibles market for 2019-2020. Whether you're buying or selling, this is the book you need.All images new for this edition. Miller's Collectibles Handbook & Price Guide 2021-2022 is the up-to-date guide to the collectables market no dealer, collector or auc
tioneer should be without. Featuring more than 4,000 objects in full color, each with a detailed description and price range, the book also shows you how to spot that rare example that may be worth twenty times more than another piece. In-depth features explain why one piece is worth more than anoth
er, show how to value an item and teach you to be your own expert when appraising everything from 20th-century glass to costume jewellery. Comprehensive sections cover a wide range of objects, with additional pages on pencils, toys, vintage handbags, trunks and costume jewellery for this edition. Ev
ery image is changed for every edition to keep the book up-to-date with collecting and buying trends. Miller's Collectibles Handbook & Price Guide 2021-2022 is the only full-color, fully-illustrated collectables price guide in the world. Judith Miller began collecting in the 1960s while a student
at Edinburgh University in Scotland. She has since extended and reinforced her knowledge of antiques through international research, becoming one of the world’s leading experts in the field. In 1979 she co-founded the international best-seller Miller’s Antiques Price Guide and has since written more
than 100 books which are held in high regard by collectors and dealers. Judith Miller appears regularly on TV and radio. She is an expert on the BBC’s Antiques Roadshow and has co-hosted the popular BBC series The House Detectives, ITV’s Antiques Trail, and Discovery’s It’s Your Bid. She has appear
ed on The Martha Stewart Show and CNN. She is a regular lecturer and contributor to numerous newspapers and magazines, including the Financial Times, the Daily Telegraph, BBC Homes & Antiques and House & Garden. She has lectured extensively, including at the V&A in London and the Smithsonian in Wash
ington.
以機器學習模型結合市場資訊之價格預測系統 - 以半導體市場為例
為了解決 spot price 的問題,作者許子敬 這樣論述:
定價策略在商業業務管理中扮演極重要的角色,越來越多的企業渴望更快速地做出最符合市場的決策,而隨著人工智慧與機器學習風潮興起,業界開始關注如何運用人工智慧與機器學習建立準確且自動化的價格預測系統。價格的波動性,在市場交易面上格外被大家重視,價格變動性相對大的產業在價格的制訂上勢必得格外謹慎,而半導體產業則屬於價格波動性相對大的產業。在半導體產業中,各家公司的定價策略就顯得十分之重要,本研究以記憶體價格為例。本研究之目的是透過機器學習演算法,開發更精準的自動化價格預測模型,而本研究提出之模型主要是運用一種機器學習模型―多層感知機(MLP Model)來進行模型的訓練,並加入十個產品共160天的歷
史價格、四個具指標性之股市資訊、以及半導體產業相關新聞三個面向市場資訊,藉此建置四個價格修正模型來改善預測結果。機器學習訓練出合適的模型特徵和調整最佳參數,透過本研究提出之修正模型,達到修正時間序列SMA模型的效果,提供更精準的價格預測,以執行更符合市場的訂價策略。從研究結果發現,對於DRAM產品線,模型一的模型修正成功率平均為57.04%;模型二的模型修正成功率平均為50.37%;模型三的模型修正成功率平均為50.37%;模型四的模型修正成功率平均為55.56%。而NAND Flash產品線,模型一的模型修正成功率平均為8.15%;模型二的模型修正成功率平均為6.67%;模型三的模型修正成功
率平均為7.41%;模型四的模型修正成功率平均為8.15%。整體而言,模型修正成功率越高,MAPE下降率也會越大。針對價格波動性較大的階段,研究結果不僅表明機器學習模型可做到記憶體的價格預測,且透過加入多種類型的市場資訊,將更能夠改善價格預測的精準度,可以提供定價策略的決策者一個準確且客觀的參考。